Introduction:
AI, or artificial intelligence, is the field of computer science that aims to create machines and systems that can perform tasks that normally require human intelligence, such as reasoning, learning, decision making, and problem solving. AI has many applications in healthcare and medicine, such as:
- Diagnosing diseases and conditions based on medical images, lab tests, symptoms, and other data
- Recommending the best treatment options for patients based on their medical history, preferences, and evidence
- Preventing diseases and health risks by predicting, monitoring, and alerting potential threats, such as chronic conditions, infectious diseases, or epidemics
- Enhancing the quality and efficiency of healthcare services by automating tasks, reducing errors, and optimizing resources
- Empowering patients and healthcare providers to access, share, and manage health information and knowledge.
Provide some examples of how AI is used to diagnose, treat and prevent diseases
- Diagnose: AI can analyse medical images, such as X-rays, CT scans, MRI scans, and ultrasound images, to detect abnormalities, such as tumour’s, fractures, or infections. For example, Google DeepMind has developed an AI system that can diagnose eye diseases from retinal scans with high accuracy and speed.
- Treat: AI can assist doctors and patients in choosing the best treatment options, such as drugs, surgery, or therapy, based on their medical history, preferences, and evidence. For example, IBM Watson Oncology can provide personalized and evidence-based recommendations for cancer treatment by analysing the patient’s genomic data, medical records, and clinical trials.
- Prevent: AI can help prevent diseases by predicting, monitoring, and alerting health risks, such as chronic conditions, infectious diseases, or epidemics. For example, Microsoft Azure Health can use AI to predict the risk of heart failure, diabetes, or stroke for a patient based on their lifestyle, biometric, and environmental data.
AI in healthcare and medicine has many benefits and challenges. Some of the benefits are:
- AI can improve the accuracy, efficiency, and accessibility of diagnosis, treatment, and prevention of diseases and health risks
- AI can provide personalized, effective, and evidence-based care for patients based on their medical history, preferences, and data
- AI can enhance the quality and efficiency of healthcare services by automating tasks, reducing errors, and optimizing resources
- AI can empower patients and healthcare providers to access, share, and manage health information and knowledge
Some of the challenges are:
- AI can pose ethical, legal, and social issues, such as privacy, security, trust, accountability, and consent
- AI can introduce potential risks, biases, and errors, such as misdiagnosis, mistreatment, or misuse of data
- AI can require high costs, skills, and infrastructure, such as data, hardware, software, and human resources
- AI can face technical and practical limitations, such as data quality, availability, and interoperability, as well as human acceptance, adoption, and collaboration.
AI can analyse medical images, lab tests, symptoms, and other data to provide accurate and timely diagnosis by using various techniques, such as:
- Machine learning: AI can learn from large amounts of labelled or unlabelled data, such as medical records, images, or texts, to find patterns, correlations, and anomalies that can help diagnose diseases and conditions. For example, AI can use supervised learning to classify images into normal or abnormal, or unsupervised learning to cluster patients into different groups based on their data.
- Deep learning: AI can use multiple layers of artificial neural networks to extract features and representations from complex and high-dimensional data, such as medical images, signals, or texts, to perform tasks such as detection, segmentation, or generation. For example, AI can use convolutional neural networks to detect tumour’s or lesions from medical images, or recurrent neural networks to generate natural language reports from medical data.
- Natural language processing: AI can use natural language processing to understand, analyse, and generate natural language texts, such as medical reports, notes, or queries, to perform tasks such as information extraction, summarization, or question answering. For example, AI can use natural language processing to extract relevant information from medical texts, such as symptoms, diagnosis, or treatment, or to answer questions from patients or doctors based on medical data.
Sure, here are some examples of AI systems for diagnosis, along with a brief description of what they do:
- IBM Watson: IBM Watson is an AI platform that can analyse various types of data, such as medical records, images, texts, and voice, to provide insights and recommendations for diagnosis and treatment. For example, IBM Watson Health can help doctors diagnose and treat cancer, diabetes, and other diseases by using natural language processing, machine learning, and computer vision.
- Google DeepMind: Google DeepMind is an AI research company that develops and applies AI to various domains, such as healthcare, gaming, and energy. For example, Google DeepMind Health can help doctors diagnose and treat eye diseases, such as diabetic retinopathy and macular degeneration, by using deep learning and computer vision to analyze retinal scans.
- Microsoft Healthcare Bot: Microsoft Healthcare Bot is an AI service that can create conversational agents, or chatbots, for healthcare applications, such as symptom checking, triage, and self-care. For example, Microsoft Healthcare Bot can help patients diagnose and treat common conditions, such as cold, flu, or headache, by using natural language processing, machine learning, and clinical protocols.
AI for diagnosis has many advantages and limitations. Some of the advantages are:
- Improved accuracy: AI can reduce human errors and biases, and increase the reliability and consistency of diagnosis, by using data-driven and evidence-based methods.
- Improved efficiency: AI can speed up the diagnosis process, and save time and resources, by automating tasks, such as image analysis, information extraction, and report generation.
Improved accessibility: AI can expand the reach and availability of diagnosis, and improve health equity and outcomes, by providing remote, low-cost, and scalable solutions, especially for underserved and rural populations.
Some of the limitations are:
- Ethical issues: AI can raise ethical questions and dilemmas, such as who is responsible for the diagnosis, how to ensure informed consent, and how to protect patient privacy and data security.
- Legal issues: AI can pose legal challenges and risks, such as how to regulate and certify AI systems, how to ensure quality and safety standards, and how to deal with liability and malpractice cases.
- Social issues: AI can create social impacts and implications, such as how to ensure fairness and transparency, how to avoid discrimination and bias, and how to foster trust and collaboration.
AI can assist doctors and patients in choosing the best treatment options, such as drugs, surgery, or therapy, by using various techniques, such as:
- Reinforcement learning: AI can learn from trial and error, and optimize the treatment policy based on the reward and feedback, such as patient outcomes, costs, and preferences. For example, AI can use reinforcement learning to find the optimal dose and schedule of chemotherapy for cancer patients, or the optimal medication and lifestyle interventions for diabetes patients.
- Generative models: AI can generate new and novel treatment options, such as drugs, molecules, or proteins, by using generative models, such as generative adversarial networks, variational autoencoders, or deep generative models. For example, AI can use generative models to design new drugs or vaccines for diseases, such as COVID-19, Alzheimer’s, or Parkinson’s.
- Decision support systems: AI can provide decision support and guidance for doctors and patients, by using decision support systems, such as expert systems, knowledge bases, or recommender systems. For example, AI can use decision support systems to suggest the best treatment options for patients based on their medical history, preferences, and evidence, or to provide explanations and recommendations for doctors based on their clinical queries.
Sure, here are some examples of AI systems for treatment, along with a brief description of what they do:
- IBM Watson Oncology: IBM Watson Oncology is an AI system that can provide personalized and evidence-based recommendations for cancer treatment by analysing the patient’s genomic data, medical records, and clinical trials. It can also help doctors and patients to compare the benefits and risks of different treatment options, such as drugs, surgery, or radiation therapy.
- Google Brain: Google Brain is an AI research team that develops and applies AI to various domains, such as healthcare, neuroscience, and robotics. For example, Google Brain has developed an AI system that can generate synthetic speech for people who have lost their voice due to diseases, such as ALS or Parkinson’s, by using deep learning and speech synthesis.
- Microsoft Inner Eye: Microsoft Inner Eye is an AI service that can help doctors and patients to plan and deliver radiotherapy for cancer treatment by using deep learning and computer vision to analyse medical images, such as CT scans or MRI scans. It can also help to reduce the time and cost of radiotherapy planning and delivery, and improve the accuracy and safety of radiotherapy.
AI for treatment has many advantages and limitations. Some of the advantages are:
- Personalized care: AI can tailor the treatment to the individual needs, preferences, and characteristics of each patient, such as their genomic data, medical history, or lifestyle factors.
- Effective care: AI can improve the effectiveness and outcomes of the treatment by using data-driven and evidence-based methods, such as clinical trials, guidelines, or best practices.
- Evidence-based care: AI can provide the latest and most relevant evidence and information for the treatment by using natural language processing, machine learning, and knowledge bases.
Some of the limitations are:
- Potential risks: AI can introduce potential risks and harms to the patient, such as adverse reactions, complications, or side effects, due to errors, inaccuracies, or uncertainties in the data, models, or algorithms.
- Biases: AI can exhibit biases and discrimination in the treatment, such as favouring or excluding certain groups of patients, due to the quality, availability, or representation of the data, models, or algorithms.
- Errors: AI can make errors and mistakes in the treatment, such as misguiding or misleading the patient or the doctor, due to the complexity, interpretability, or explain ability of the data, models, or algorithms.
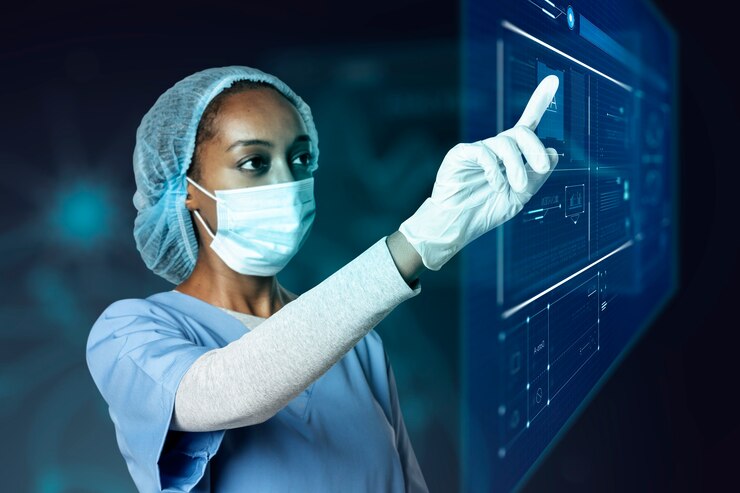
AI can help prevent diseases by predicting, monitoring, and alerting health risks, such as chronic conditions, infectious diseases, or epidemics, by using various techniques, such as:
- Predictive analytics: AI can use predictive analytics to forecast the future occurrence, severity, and spread of diseases and health risks, based on historical and current data, such as epidemiological, demographic, or environmental data. For example, AI can use predictive analytics to estimate the risk of developing heart disease, diabetes, or cancer for a patient, or to predict the outbreak and transmission of diseases, such as COVID-19, influenza, or malaria.
- Monitoring and surveillance: AI can use monitoring and surveillance to track and measure the status, trends, and patterns of diseases and health risks, based on real-time and continuous data, such as wearable, sensor, or social media data. For example, AI can use monitoring and surveillance to monitor the vital signs, symptoms, and behaviours of patients, or to detect and report the occurrence and location of diseases, such as Ebola, Zika, or dengue.
- Alerting and intervention: AI can use alerting and intervention to notify and advise the patients, doctors, and public health authorities about the diseases and health risks, and to suggest and implement the appropriate actions and measures, such as prevention, treatment, or containment. For example, AI can use alerting and intervention to alert the patients and doctors about the need for screening, testing, or medication, or to recommend and enforce the best practices, such as social distancing, quarantine, or vaccination.
Sure, here are some examples of AI systems for prevention, along with a brief description of what they do:
- Apple HealthKit: Apple HealthKit is an AI service that can integrate and manage health and fitness data from various sources, such as apps, devices, or medical records, and provide insights and recommendations for prevention and wellness. For example, Apple HealthKit can help users to track and improve their physical activity, sleep, nutrition, or heart health, by using machine learning, sensor data, and health metrics.
- Google Fit: Google Fit is an AI service that can measure and monitor health and fitness data from various sources, such as apps, devices, or wearables, and provide insights and recommendations for prevention and wellness. For example, Google Fit can help users to achieve their health and fitness goals, such as steps, calories, or heart points, by using machine learning, sensor data, and gamification.
- Microsoft Azure Health: Microsoft Azure Health is an AI service that can analyse and predict health and disease risks from various sources, such as biometric, lifestyle, or environmental data, and provide insights and recommendations for prevention and intervention. For example, Microsoft Azure Health can help users to prevent and manage chronic conditions, such as heart failure, diabetes, or stroke, by using machine learning, cloud computing, and health analytics.
AI for prevention has many advantages and limitations. Some of the advantages are:
- Proactive health: AI can help users to take charge of their own health and wellness, and to prevent or delay the onset of diseases and health risks, by providing personalized and timely feedback, guidance, and motivation.
- Preventive health: AI can help users to avoid or reduce the severity and complications of diseases and health risks, by providing early detection, diagnosis, and intervention, and by suggesting the best practices and measures, such as screening, testing, or vaccination.
- Participatory health: AI can help users to engage and collaborate with their healthcare providers and communities, and to share and access health information and knowledge, by providing interactive and social platforms, such as chatbots, apps, or networks.
Some of the limitations are:
- Privacy issues: AI can pose privacy threats and challenges, such as how to protect and control the personal and sensitive health data of the users, and how to ensure the consent and awareness of the users, when collecting, storing, and processing the data, especially from third-party sources, such as apps, devices, or wearables.
- Security issues: AI can pose security risks and vulnerabilities, such as how to prevent and defend against the unauthorized access, use, or disclosure of the health data of the users, and how to ensure the integrity and reliability of the data, especially from malicious attacks, such as hacking, phishing, or spoofing.
- Trust issues: AI can pose trust issues and barriers, such as how to establish and maintain the trust and confidence of the users in the AI systems, and how to ensure the transparency and accountability of the AI systems, especially when the systems make decisions or recommendations that affect the health and well-being of the users.
Conclusion
AI is a powerful and promising technology that can transform healthcare and medicine by providing new and innovative ways to diagnose, treat, and prevent diseases and health risks. AI can improve the accuracy, efficiency, and accessibility of diagnosis, treatment, and prevention, and provide personalized, effective, and evidence-based care for patients and healthcare providers. However, AI also poses many challenges and limitations, such as ethical, legal, and social issues, potential
risks, biases, and errors, and technical and practical barriers. Therefore, it is important to ensure that AI is developed and applied in a responsible, ethical, and human-cantered manner, and that it is aligned with the values, needs, and preferences of the users and stakeholders. AI is not a replacement, but a complement, to human intelligence, expertise, and judgment, and it requires collaboration and cooperation among various disciplines, sectors, and communities. AI has the potential and the responsibility to make healthcare and medicine better, safer, and more accessible for everyone.
8 thoughts on “The Future of Healthcare and Medicine with AI”