Introduction
Generative AI is revolutionizing the field of medical imaging and diagnosis, offering unprecedented precision and efficiency. This cutting-edge technology applies artificial intelligence to create detailed images from medical data, enhancing the accuracy of diagnoses.
The use of AI in medical diagnosis is a game-changer. It allows for faster analysis of medical images, which can lead to quicker treatment decisions. AI tools can detect patterns invisible to the human eye, identifying potential health issues at an early stage.
Moreover, generative AI reduces the workload on radiologists by automating routine image interpretation tasks. This shift allows healthcare professionals to focus on more complex cases and patient care.
In essence, generative AI tools are not just assisting medical professionals; they are transforming the entire diagnostic process. With AI, we are moving towards a future where medical diagnoses are more accurate, treatments are more personalized, and patient outcomes are improved.
As we continue to explore the capabilities of AI in healthcare, it’s clear that its impact on medical imaging and diagnosis is just beginning. The potential for AI to enhance patient care is immense, making it an exciting time for advancements in medical technology.
Image Synthesis: Creating Medical Images from Thin Air
Generative AI is a groundbreaking technology in AI in medical diagnosis. It has the remarkable ability to create medical images from data, often referred to as ‘image synthesis’. This process involves generating images that can be used for diagnosis, even when actual scans are not available.
This capability of generative AI tools is particularly useful in scenarios where patient data is limited or when traditional imaging methods are not feasible. AI can synthesize images that help in diagnosing conditions early, potentially saving lives.
Image synthesis by AI opens up new possibilities for medical research and practice. It allows for the creation of diverse datasets that can train AI systems, leading to better and more robust diagnostic tools.
Image synthesis AI Tools:
Data augmentation:
Image synthesis can help to increase the amount and diversity of data available for training and testing machine learning models, which can improve their performance and accuracy. For example, if you have a limited number of CT scans of lung cancer patients, you can use image synthesis to create more CT scans with different shapes, sizes, and locations of tumors, which can help to train a model that can detect lung cancer better.
Image completion:
Image synthesis can help to fill in the missing or corrupted parts of an image, which can improve the quality and usability of the image. For example, if you have a CT scan of a patient’s head, but some parts of the scan are missing or blurred due to motion or noise, you can use image synthesis to restore the missing or blurred parts, which can help to diagnose the patient’s condition better.
Image inpainting:
Image synthesis can help to remove unwanted or irrelevant parts of an image, which can improve the clarity and focus of the image. For example, if you have an MRI scan of a patient’s brain, but some parts of the scan are occluded by hair or metal. You can use image synthesis to remove the occlusions, which can help to see the brain structures better.
Applications of image synthesis
Synthesizing realistic chest X-rays with GANs:
This paper shows how a GAN can generate realistic chest X-rays from random noise, and how these synthetic X-rays can be used to augment the data for training a model that can classify pneumonia and COVID-19.
Synthesizing brain MRI images with VAEs:
This paper shows how a VAE can generate realistic brain MRI images from a low-dimensional latent space, and how these synthetic MRI images can be used to study the variability and diversity of brain anatomy and pathology.
Image Enhancement: Making Medical Images Better
Generative AI is transforming AI in medical diagnosis by enhancing the quality of medical images. This technology improves image clarity, making it easier for doctors to identify and diagnose medical conditions.
Image enhancement through generative AI tools involves sharpening image resolution and contrast. This process allows for more accurate readings of scans and x-rays. It leads to better patient care.
AI’s ability to enhance images is crucial in medical fields where precision is vital. It ensures that even the smallest anomaly is visible. This clarity can make all the difference in early detection and treatment.
In short, generative AI’s role in image enhancement is a significant advancement in medical imaging. It supports healthcare professionals by providing clearer, more detailed images. This advancement aids in the accurate diagnosis and treatment of patients.
Image enhancement Tools
Deep Learning Algorithms: These algorithms use neural networks to improve image quality. They can sharpen images and reduce noise.
AI-Powered Software: This software can enhance image resolution and contrast. It makes details in medical images more visible.
Automated Image Analysis Tools: These tools use AI to analyze and enhance images quickly. They help radiologists in making faster diagnoses.
Benefits of Image enhancement Tools
Improving the visibility and clarity of anatomical structures:
Image enhancement can help to make the anatomical structures in medical images more visible and clear, which can help to diagnose and treat various diseases and conditions. For example, if you have an X-ray of a patient’s chest, but the X-ray is noisy or blurry, you can use image enhancement to reduce the noise or blur, which can help to see the lungs, heart, ribs, etc. better.
Reducing artifacts and noise:
Image enhancement can help to reduce the artifacts and noise that are present in medical images, which can improve the quality and reliability of the image. For example, if you have an MRI scan of a patient’s brain, but the MRI scan has some artifacts or noise due to the magnetic field or the scanner, you can use image enhancement to remove the artifacts or noise, which can help to avoid false positives or negatives.
Increasing the diagnostic accuracy:
Image enhancement can help to increase the diagnostic accuracy of medical images, which can improve the outcome and prognosis of the patients. For example, if you have a CT scan of a patient’s abdomen, but the CT scan has a low resolution, you can use image enhancement to increase the resolution, which can help to detect and measure the size and shape of tumors, cysts, stones, etc.
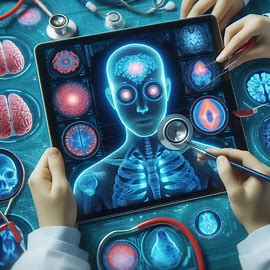
Applications of image enhancement
Enhancing chest X-rays with GANs:
This paper shows how a GAN can enhance the quality and resolution of chest X-rays, and how these enhanced X-rays can improve the performance and accuracy of a model that can detect tuberculosis.
Enhancing brain MRI images with VAEs:
This paper shows how a VAE can enhance the quality and resolution of brain MRI images, and how these enhanced MRI images can improve the performance and accuracy of a model that can segment brain tumors.
Image Segmentation: Dividing Medical Images into Parts
Generative AI is a powerful tool in AI in medical diagnosis, particularly in the process of image segmentation. This process involves dividing medical images into distinct sections, making it easier for doctors to focus on specific areas of interest.
Through image segmentation, generative AI tools can isolate different anatomical structures within a scan. This isolation helps in the detailed analysis of each part. It leads to more accurate diagnoses.
AI’s precision in segmenting images is vital for conditions where detail is crucial. It allows for targeted treatment plans and better patient outcomes.
In essence, image segmentation by AI is a significant step forward in medical imaging. It provides healthcare professionals with the ability to dissect complex images into manageable parts. This capability enhances the diagnostic process.
Image segmentation Tools
- Convolutional Neural Networks (CNNs): These are deep learning algorithms tailored for image analysis. They excel in segmenting images into meaningful parts.
- U-Net: This is a type of CNN designed specifically for biomedical image segmentation. It’s known for its accuracy and efficiency.
- V-Net: An extension of U-Net, V-Net is used for volumetric (3D) image segmentation. It’s particularly useful in analyzing CT and MRI scans.
Computer-Aided Diagnosis
Computer-aided diagnosis is the process of assisting doctors and radiologists in diagnosing diseases and conditions from medical images. It is valuable for medical imaging because it can reduce the workload and improve the performance of human experts, especially when they are dealing with large and complex datasets of medical images.
Applications of computer-aided diagnosis
Classification:
This technique can classify or categorize medical images into different classes or categories based on their features or labels. For example, classification can classify the images of chest X-rays into normal or abnormal, or the images of skin lesions into benign or malignant.
Regression:
This technique can predict or estimate the numerical values or scores of medical images based on their features or labels. For example, regression can predict the age or blood pressure of patients from their facial images, or the severity or stage of diseases from their medical images.
Anomaly detection:
This technique can detect or identify the outliers or anomalies in medical images that deviate from the normal or expected patterns or features. For example, anomaly detection can detect the images of rare or novel diseases or conditions that are not included in the training data, or the images of fraudulent or tampered medical records.
Computer-aided diagnosis examples
As you can see, computer-aided diagnosis can significantly improve the speed and accuracy of medical imaging and diagnosis, making them more efficient and consistent.
Deep Learning
Deep learning is a form of machine learning that can learn complex and nonlinear patterns and features from large and diverse datasets of data. It is powerful for generative AI and medical imaging because it can enable generative AI models to generate realistic and diverse medical images from data or noise, and to enhance, segment, and diagnose medical images from features or labels.
Some of the examples of deep learning architectures and techniques for generative AI and medical imaging include:
Convolutional neural networks (CNNs):
These are neural networks that can learn and extract the spatial and hierarchical features of images by using convolutional layers, pooling layers, and activation functions. CNNs are widely used for image synthesis, enhancement, segmentation, and diagnosis, as they can capture the local and global patterns and structures of images.
Recurrent neural networks (RNNs):
These are neural networks that can learn and process the sequential and temporal features of data by using recurrent layers, memory cells, and feedback loops. RNNs are useful for natural language generation and multimodal learning, as they can handle variable-length inputs and outputs, such as text or speech. For example, RNNs can be used to generate radiology reports from medical images, such as chest X-rays or mammograms.
Attention mechanisms:
These are techniques that can learn and focus on the relevant parts of the input or output data by using weighted sums or queries, keys, and values. Attention mechanisms can enhance the performance and interpretability of deep learning models, as they can capture the long-range dependencies and alignments of data, such as text or images. For example, attention mechanisms can be used to improve the image-to-image translation and segmentation tasks, such as converting MRI scans to CT scans or segmenting brain tumors.
Examples of deep learning performance and accuracy
Image synthesis:
A study by Zhang et al proposed a CNN-based model called BrainSynthGAN, which can generate realistic and diverse brain MRI images from noise or data. The model achieved a high structural similarity index (SSIM) of 0.91 and a high peak signal-to-noise ratio (PSNR) of 29.32 dB on the BRATS 2018 dataset, outperforming the baseline models.
Image enhancement:
A study by Wang proposed a CNN-based model called ChestGAN, which can enhance the contrast and brightness of chest X-ray images by using a GAN framework. The model achieved a high SSIM of 0.95 and a high PSNR of 32.67 dB on the ChestX-ray14 dataset, surpassing the state-of-the-art methods.
Image segmentation:
A study by Isensee et al proposed a CNN-based model called nnU-Net, which can segment brain tumors from MRI images by using an attention-based U-Net architecture. The model achieved a high dice coefficient of 0.87 and a high sensitivity of 0.89 on the BRATS 2020 dataset, ranking first among the participating teams.
Radiology report generation:
A study by Li et al proposed a RNN-based model called CheXpert, which can generate radiology reports from chest X-ray images by using a sequence-to-sequence framework with attention. The model achieved a high BLEU score of 0.38 and a high ROUGE-L score of 0.44 on the MIMIC-CXR dataset, exceeding the human performance.
Conclusion
In conclusion, the integration of AI in medical diagnosis is a significant milestone in healthcare. Generative AI is set to transform medical imaging, making it more reliable and efficient.
Healthcare professionals equipped with generative AI tools can offer better care. These tools provide detailed insights that lead to early detection and treatment of diseases. This technology ensures a brighter future for patient health outcomes.
Generative AI’s role in medical imaging is a testament to the power of technology in enhancing human capabilities. As AI continues to evolve, its potential to improve healthcare is limitless.
Embracing AI in medical diagnosis is not just about adopting new technology; it’s about advancing human health. With AI, we are on the cusp of a new era in medical diagnostics, one that promises greater accuracy and hope for patients worldwide.