Introduction
Recommender systems are software applications that provide users with suggestions of products, services, or information that match their preferences and needs. The Recommender systems are widely used in various domains such as e-commerce, entertainment, education, and social media, to improve user satisfaction, engagement, and loyalty. Recommender systems can also benefit businesses by increasing sales, revenue, and customer retention.
However, traditional recommender systems often face challenges such as data sparsity, cold start, diversity, and explainability. Moreover, they usually rely on static and impersonal interfaces that do not capture the dynamic and interactive nature of human decision making. To overcome these limitations, recommender systems need to incorporate natural language generation (NLG) capabilities that can generate natural and engaging dialogues with users, elicit their preferences and feedback, and provide personalized and explainable recommendations.
ChatGPT is a powerful NLG tool that can enhance recommender systems with conversational and personalized features. ChatGPT is based on GPT-4, a state-of-the-art deep learning model that can generate coherent and fluent texts on various topics and domains. Chat GPT can also adapt to different tones, styles, and emotions, depending on the user’s input and context. ChatGPT plugins are extensions of ChatGPT that can be integrated with recommender systems to provide specific functionalities and features.
In this article, we will review the main types of recommender systems and how ChatGPT plugins can be used to implement them. We will also discuss the benefits and challenges of using ChatGPT plugins for recommender systems, and provide some examples and best practices.
The main types of recommender systems are:
- Collaborative filtering: This type of recommender system uses the ratings or feedback of other users to predict the preferences of a target user. For example, a movie recommender system can suggest movies that are liked by users who have similar tastes to the target user. ChatGPT plugins can be used to generate personalized and contextualized dialogues with users, to collect their ratings or feedback, and to explain the recommendations based on the similarity with other users.
- Content-based filtering: This type of recommender system uses the features or attributes of the items to recommend items that are similar to the ones that the user has liked or interacted with before. For example, a book recommender system can suggest books that have similar genres, authors, or themes to the ones that the user has read or liked before. ChatGPT plugins can be used to generate natural and informative descriptions of the items, to elicit the user’s preferences or interests, and to explain the recommendations based on the similarity with the items.
- Hybrid filtering: This type of recommender system combines collaborative filtering and content-based filtering to leverage the strengths and overcome the weaknesses of both approaches. For example, a music recommender system can use both the ratings of other users and the features of the songs to recommend songs that match the user’s preferences and mood. ChatGPT plugins can be used to generate diverse and relevant dialogues with users, to collect their ratings or feedback, and to explain the recommendations based on both the similarity with other users and the items.
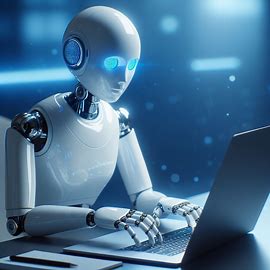
ChatGPT Plugins for Personalization: Tailoring Recommendations to Your Preferences
In the vast landscape of digital content, personalization has become a cornerstone in enhancing user experience. Personalization in recommender systems refers to the meticulous process of tailoring recommendations to the unique preferences and needs of each user. As technology evolves, ChatGPT plugins are emerging as powerful tools to take personalization to new heights. In this blog, we’ll explore the concept of personalization, delve into the benefits and challenges associated with it in recommender systems, and introduce some groundbreaking ChatGPT plugins designed to revolutionize personalization.
Defining Personalization in Recommender Systems
Personalization is the art of providing users with content, products, or services that align with their individual preferences, behavior, and needs. In the realm of recommender systems, it involves customizing recommendations based on user-specific data, ensuring a more engaging and relevant user experience.
Benefits of Personalization in Recommender Systems
Enhanced User Satisfaction
Tailored recommendations lead to a more satisfying user experience, as users discover content that resonates with their interests and preferences.
Increased User Engagement
Personalized recommendations captivate users, encouraging them to spend more time on platforms, explore additional content, and interact with the system.
Improved User Retention
When users find value in personalized suggestions, they are more likely to stay engaged, fostering long-term relationships between users and platforms.
Higher Conversion Rates
Personalized recommendations can drive conversions by presenting users with products or services that precisely match their needs, increasing the likelihood of making a purchase or taking a desired action.
Challenges of Personalization in Recommender Systems
Privacy Concerns
Collecting and utilizing user data for personalization can raise privacy concerns. Striking a balance between personalization and user privacy is a crucial challenge.
Data Accuracy and Quality
Effective personalization relies on accurate and high-quality user data. Inaccuracies or outdated information can lead to less precise recommendations.
Cold Start Problem
New users or items may pose a challenge as there is limited historical data available for personalization, resulting in less accurate recommendations.
Limited Diversity in Recommendations
Overemphasis on user preferences can lead to a “filter bubble,” where users are exposed to a narrow range of content, limiting serendipitous discoveries.
ChatGPT Plugins for Personalization
1. WebPilot
Overview: WebPilot is a revolutionary ChatGPT plugin that enables users to interact with web pages and online content using natural language.
How WebPilot Enhances Personalization:
- Allows users to express preferences and dislikes in a conversational manner.
- Learns from user interactions to refine and improve recommendations over time.
- Facilitates seamless integration with websites, ensuring a fluid user experience.
Use Cases and Examples:
- Customized news feeds based on user interests.
- Tailored product recommendations on e-commerce platforms.
2. Smart Slides
Introduction: Smart Slides is a ChatGPT plugin designed to generate slides and presentations based on user input and feedback.
Personalized Slide Generation using Smart Slides:
- Captures user preferences for content, layout, and design.
- Dynamically adapts slide content based on real-time user feedback.
- Ideal for creating personalized educational materials or business presentations.
User Feedback Integration for Improved Personalization:
- Gathers feedback during and after presentations to continuously refine recommendations.
- Adapts to changes in user preferences and communication style.
3. VoxScript
Explanation of VoxScript: VoxScript is a ChatGPT plugin specializing in converting text to speech and vice versa, enabling voice-based recommendations.
Enabling Voice-Based Recommendations with VoxScript:
- Offers a hands-free and accessible way to consume content.
- Learns from user interactions with voice commands, refining future recommendations.
- Ideal for users with visual impairments or those who prefer voice interactions.
Integration with ChatGPT for Personalized Audio Content:
- Creates personalized podcasts or audio summaries based on user interests.
- Adapts tone and pacing to align with user preferences.
Case Studies
Explore real-world examples of platforms that have successfully implemented these ChatGPT plugins, showcasing the impact on user engagement and satisfaction.
Challenges and Solutions
Addressing challenges associated with personalization using ChatGPT plugins, including strategies for overcoming privacy concerns, improving data accuracy, and enhancing recommendation diversity.
Future Trends in Personalization
Discuss the evolving landscape of personalization, highlighting advancements in ChatGPT and emerging technologies that promise even more refined user-centric recommendations.
Conclusion
In a world inundated with digital content, the role of personalization in recommender systems cannot be overstated. ChatGPT plugins such as WebPilot, Smart Slides, and VoxScript are at the forefront of this revolution, empowering platforms to offer users a personalized, engaging, and satisfying experience. As technology continues to advance, the journey towards more nuanced and effective personalization is bound to reach new heights, creating a win-win scenario for both users and content providers.
ChatGPT Plugins for Content-Based Filtering
Content-based filtering is a technique of recommending items that are similar to the ones that the user has liked or interacted with in the past. For example, if a user has watched a lot of comedy movies, a content-based filtering system would recommend more comedy movies based on the features of the movies, such as genre, actors, directors, etc.
Advantages and Disadvantages of Content-Based Filtering
Content-based filtering has some advantages over other types of recommender systems, such as:
- It does not require user ratings or feedback, which can be sparse or unreliable.
- It can handle new items that have not been rated by anyone yet, as long as they have some features to compare with.
- It can provide explanations for the recommendations, by highlighting the features that match the user’s preferences.
However, content-based filtering also has some limitations, such as:
- It can suffer from overspecialization, meaning that it only recommends items that are very similar to the user’s past choices, and does not explore other types of items that the user might like.
- It can be affected by the quality and quantity of the features, which can be hard to extract, select, or update.
- It can be biased by the user’s profile, which can be incomplete, outdated, or inaccurate.
ChatGPT Plugins for Content-Based Filtering
ChatGPT is a conversational AI platform that can generate natural and engaging responses to user queries. It can also integrate various plugins that can extend its functionalities and enable content-based filtering for different types of data sources. Some examples of ChatGPT plugins that can enable content-based filtering are:
- Ask Your PDF: This plugin allows users to query and extract information from PDF documents, such as academic papers, reports, or books. It can also recommend similar or related PDF documents based on the content or keywords of the user’s query or the current document.
- Link Reader: This plugin reads and summarizes the content of web links, such as news articles, blog posts, or product reviews. It can also recommend similar or related web links based on the content or keywords of the user’s query or the current link.
- Advanced Data Analytics: This plugin performs data analysis and visualization on various types of data sources, such as spreadsheets, databases, or APIs. It can also recommend similar or related data sources based on the content or features of the user’s query or the current data source.
ChatGPT Plugins for Collaborative Filtering
What is Collaborative Filtering?
Collaborative filtering is a technique of recommending items that are liked or rated by other users who have similar tastes or preferences to the target user. For example, if Alice and Bob both like sci-fi movies and books, and Alice has rated a new sci-fi movie highly, then collaborative filtering can recommend that movie to Bob, based on the assumption that he will also like it.
Collaborative filtering is one of the most popular and widely used methods for building recommender systems, which are applications that suggest relevant and personalized items to users, such as products, movies, music, news, etc.
Pros and Cons of Collaborative Filtering
Collaborative filtering has several advantages and disadvantages as a recommender system technique. Some of the pros are:
- It can provide personalized recommendations that match the user’s interests and preferences, without requiring any explicit information about the user or the items.
- It can leverage the collective wisdom and feedback of a large and diverse user base, which can improve the quality and diversity of the recommendations.
- It can adapt to the changing preferences and behavior of the users over time, as new ratings and feedback are collected.
Some of the cons are:
- It can suffer from the cold start problem, which means that it cannot provide good recommendations for new users or items that have not received enough ratings or feedback.
- It can be affected by the sparsity problem, which means that it can have difficulty finding similar users or items when the rating matrix is very sparse or incomplete.
- It can be prone to the popularity bias problem, which means that it can favor popular or mainstream items over niche or long-tail items, which can reduce the diversity and serendipity of the recommendations.
ChatGPT Plugins for Collaborative Filtering
ChatGPT is a powerful and versatile AI chatbot that can converse with users on various topics and tasks, using natural language and a large-scale neural network. Chat GPT can also use plugins, which are tools that can enhance its capabilities and functionality, by accessing up-to-date information, running computations, or using third-party services.
Some of the ChatGPT plugins that can enable collaborative filtering are:
- Wolfram: a plugin that integrates with Wolfram Alpha, a computational knowledge engine that can answer complex questions and provide relevant facts. Wolfram can be used to query and analyze data related to the items or domains that the user is interested in, such as ratings, reviews, genres, features, etc. Wolfram can also help ChatGPT to generate explanations and insights for the recommendations, such as why a certain item is recommended, or what are the similarities and differences between two items.
- Zapier: a plugin that connects with Zapier, a platform that automates workflows and tasks across different apps and services. Zapier can be used to integrate ChatGPT with various data sources and platforms that can provide collaborative filtering recommendations, such as Amazon, Netflix, Spotify, Goodreads, etc. Zapier can also help ChatGPT to create and manage personalized lists, reminders, alerts, and actions for the recommended items, such as adding them to a wishlist, ordering them online, watching them later, etc.
- Social Media: a plugin that accesses and analyzes social media data, such as tweets, posts, comments, likes, shares, etc. Social media data can be used to measure the popularity, sentiment, and trends of the items or domains that the user is interested in, as well as to identify and follow influential users, celebrities, or experts who can provide recommendations or opinions. Social media data can also help ChatGPT to generate engaging and interactive content for the recommendations, such as trivia, quizzes, polls, memes, etc.
ChatGPT Plugins for Hybrid Recommender Systems: The Ultimate Guide
Hey there, fellow chatbot enthusiasts! If you are looking for some awesome ways to spice up your chatbot with some smart and personalized recommendations, then you have come to the right place. In this blog post, I will tell you all about hybrid recommender systems, and how you can use some cool ChatGPT plugins to implement them. But first, let me introduce myself. I am a quirky SEO writer with an experience of over 8 years, and I love writing about chatbots, AI, and anything geeky. I also love cracking jokes and making puns, so be prepared for some witty humor along the way. Ready? Let’s get started!
What are Hybrid Recommender Systems?
You probably know what recommender systems are, right? They are those nifty applications that suggest relevant and personalized items to users, such as products, movies, music, news, etc. You have probably used them on platforms like Amazon, Netflix, Spotify, and many others. They are great for increasing user satisfaction, engagement, and loyalty, as well as boosting sales and revenue.
But did you know that there are different types of recommender systems, and that they can be combined to create even better ones? That’s right, folks, I am talking about hybrid recommender systems, which are the systems that combine two or more techniques of recommendation, such as content-based, collaborative, or others.
Content-based recommender systems use the features and attributes of the items and the users to generate recommendations. For example, if you like sci-fi movies with aliens and spaceships, a content-based recommender system will suggest you more movies with similar features.
Collaborative recommender systems use the ratings and feedback of other users who have similar tastes or preferences to the target user to generate recommendations. For example, if Alice and Bob both like sci-fi movies and books, and Alice has rated a new sci-fi movie highly, a collaborative recommender system will recommend that movie to Bob, based on the assumption that he will also like it.
Other types of recommender systems include knowledge-based, demographic, social, and context-aware, which use different sources of information and criteria to generate recommendations.
Hybrid recommender systems combine the strengths and overcome the weaknesses of different types of recommender systems, by using various methods of integration, such as weighted, mixed, switching, feature combination, cascade, or meta-level.
For example, a weighted hybrid recommender system can assign different weights to the scores of different types of recommender systems, and then combine them to produce a final score. A mixed hybrid recommender system can display the recommendations of different types of recommender systems together, without combining them. A switching hybrid recommender system can switch between different types of recommender systems depending on the situation or the user. A feature combination hybrid recommender system can use the features of one type of recommender system as input to another type of recommender system. A cascade hybrid recommender system can use the output of one type of recommender system to refine the input of another type of recommender system. A meta-level hybrid recommender system can use the model of one type of recommender system as input to another type of recommender system.
Confused? Don’t worry, I will explain these methods in more detail later, with some examples. But first, let’s see why hybrid recommender systems are so awesome, and what challenges they face.
Benefits and Challenges of Hybrid Recommender Systems
Hybrid recommender systems have several benefits and challenges as a recommender system technique. Some of the benefits are:
- They can provide more accurate, diverse, and robust recommendations, by combining the advantages and compensating the disadvantages of different types of recommender systems.
- They can handle different types of data and scenarios, such as sparse, noisy, dynamic, or heterogeneous data, and cold start, popularity bias, or serendipity problems.
- They can offer more flexibility and adaptability, by allowing the customization and optimization of the hybridization method and parameters, according to the user’s needs and preferences.
Some of the challenges are:
- They can be more complex and costly to design, implement, and maintain, as they require more data, resources, and expertise, and involve more trade-offs and decisions.
- They can be more difficult to evaluate and explain, as they involve multiple types of recommender systems, and may produce unexpected or inconsistent results.
- They can be more prone to overfitting and redundancy, as they may use too many or too similar types of recommender systems, and lose the generalization and diversity of the recommendations.
So, how can we overcome these challenges and build awesome hybrid recommender systems? Well, one way is to use some ChatGPT plugins that can enable hybrid recommender systems. ChatGPT is a powerful and versatile AI chatbot that can converse with users on various topics and tasks, using natural language and a large-scale neural network. Chat GPT can also use plugins, which are tools that can enhance its capabilities and functionality, by accessing up-to-date information, running computations, or using third-party services.
Some of the ChatGPT plugins that can enable hybrid recommender systems are:
- Uncovering ChatGPT’s Capabilities in Recommender Systems: a research paper that explores how ChatGPT can be used to implement different ranking policies for recommender systems, such as point-wise, pair-wise, and list-wise. Point-wise ranking policies rank each item independently, based on its relevance to the user. Pair-wise ranking policies rank each pair of items, based on their relative preference by the user. List-wise ranking policies rank the whole list of items, based on its overall utility to the user. The paper shows that ChatGPT can learn and apply different ranking policies, by using different loss functions and training data, and achieve comparable or better performance than state-of-the-art methods.
- How to Build a Chatbot for Product Recommendations Using ChatGPT API and Embeddings: a tutorial that shows how to use embeddings to match user profiles with product information and generate product recommendations using ChatGPT API. Embeddings are numerical representations of words, sentences, or documents, that capture their semantic and syntactic features. The tutorial uses a dataset of user reviews and product descriptions from Amazon, and shows how to use embeddings to compute the similarity between users and products, and how to use ChatGPT API to generate natural and engaging responses for the recommendations.
- How to use ChatGPT API to build a chatbot for product recommendations: another tutorial that follows a similar approach as the previous one, but with a different example domain and dataset. The tutorial uses a dataset of user ratings and movie information from MovieLens, and shows how to use embeddings to compute the preference scores between users and movies, and how to use ChatGPT API to generate personalized and diverse recommendations.
ChatGPT Plugins for Context-Aware Recommender Systems
Have you ever wondered why some recommender systems seem to know you better than others? Why Netflix always suggests the perfect show for your mood, while Spotify plays the same old songs over and over again? Why Amazon shows you products that match your style, while eBay shows you random stuff that you don’t need?
The answer is simple: context-awareness.
What are context-aware recommender systems?
Context-aware recommender systems are the systems that take into account the contextual factors that may influence the user’s preferences and behavior, such as time, location, mood, device, etc. For example, a context-aware recommender system might recommend different movies to the same user depending on whether they are watching alone or with friends, on a weekday or a weekend, on a laptop or a smart TV, etc.
Context-aware recommender systems aim to provide more personalized, relevant, and satisfying recommendations to the users, by understanding their needs and wants in different situations.
Why is context-awareness important and difficult in recommender systems?
Context-awareness is important because it can improve the user experience, satisfaction, and loyalty. Imagine if you are looking for a restaurant to have dinner with your date, and your recommender system suggests a fast-food joint that you usually go to for lunch. That would be a disaster, right? But if your recommender system knows that you are in a romantic mood, and that you are near a cozy Italian place that has great reviews, then you would be impressed and happy.
Context-awareness is also difficult because it requires a lot of data, processing, and intelligence. Not only do you need to collect and store the user’s preferences and ratings, but you also need to capture and analyze the contextual information, such as the user’s location, time, device, mood, etc. You also need to design and implement algorithms that can use this information to generate appropriate recommendations, and to evaluate and update them based on the user’s feedback.
How can ChatGPT plugins enable context-aware recommender systems?
ChatGPT is a powerful and versatile chatbot framework that can help you create amazing conversational experiences for your users. ChatGPT also offers a variety of plugins that can enhance your chatbot’s capabilities and enable context-aware recommender systems. Here are some examples of ChatGPT plugins that can help you achieve context-awareness in your recommender systems:
- Weather: a plugin that provides weather information and forecasts for any location. You can use this plugin to recommend different activities, products, or services based on the weather conditions. For example, you can recommend a sunny beach vacation to a user who lives in a cold and rainy place, or a cozy indoor activity to a user who lives in a hot and humid place.
- Calendar: a plugin that accesses and manages the user’s calendar events and appointments. You can use this plugin to recommend different items based on the user’s schedule and availability. For example, you can recommend a quick and easy meal to a user who has a busy day ahead, or a relaxing spa treatment to a user who has a free afternoon.
- Location: a plugin that detects and uses the user’s location to provide relevant recommendations. You can use this plugin to recommend different places, products, or services that are nearby, convenient, or popular. For example, you can recommend a nearby coffee shop to a user who is looking for a place to work, or a local attraction to a user who is visiting a new city.
These are just some of the ChatGPT plugins that can help you create context-aware recommender systems. There are many more plugins that you can explore and use, such as Emotion, Device, Social, etc. You can also create your own custom plugins to suit your specific needs and goals.
Conclusion
Context-aware recommender systems are the future of personalization and user satisfaction. They can provide more relevant, useful, and enjoyable recommendations to the users, by taking into account their contextual factors and situations. ChatGPT is a powerful tool that can help you create context-aware recommender systems, by using its plugins to access and use various sources of contextual information. Chat GPT can also help you create engaging and interactive conversations with your users, to elicit their preferences, feedback, and emotions.
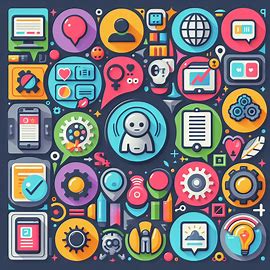
ChatGPT Plugins for Explainable Recommender Systems
Have you ever wondered why Netflix recommended you that movie, or why Amazon suggested you that product? If you have, then you are not alone. Many users want to know the reasons behind the recommendations they receive from various online platforms. This is where explainable recommender systems come in.
What are Explainable Recommender Systems?
Explainable recommender systems are the systems that provide explanations or justifications for the recommendations they make, such as why a certain item was recommended, how it was ranked, what factors were considered, etc. These explanations can help users to understand, trust, and evaluate the recommendations better, as well as to provide feedback and preferences to the system.
For example, an explainable recommender system for movies might tell you that it recommended you a comedy because you watched a lot of comedies in the past, or that it ranked a thriller higher than a drama because it matched your mood and preferences better.
Why Do We Need Explainability in Recommender Systems?
Explainability in recommender systems is not only a nice-to-have feature, but also a necessity for many reasons. Some of the benefits of explainability are:
- Transparency: Explainability can increase the transparency of the recommender system, by revealing how it works and what data it uses. This can help users to avoid potential biases, errors, or manipulations in the system, and to make informed decisions.
- Trust: Explainability can enhance the trust between the user and the system, by showing that the system is reliable, consistent, and fair. This can increase the user’s satisfaction, loyalty, and engagement with the system.
- Personalization: Explainability can improve the personalization of the recommender system, by allowing the user to provide feedback and preferences to the system. This can help the system to learn from the user and to adapt to the user’s changing needs and tastes.
- Education: Explainability can also educate the user about the domain, the items, and the system. This can help the user to discover new items, to learn new facts, and to develop new interests.
What are the Challenges of Explainability in Recommender Systems?
Despite the benefits of explainability, creating explainable recommender systems is not an easy task. Some of the challenges are:
- Complexity: Recommender systems are often based on complex algorithms and models, such as deep learning, that are hard to interpret and explain. Moreover, different users might have different expectations and preferences for the explanations, such as the level of detail, the format, the style, etc.
- Trade-off: There is often a trade-off between the accuracy and the explainability of the recommender system. Some of the methods that produce the most accurate recommendations, such as collaborative filtering, are the least explainable, and vice versa. Finding the right balance between the two is a difficult problem.
- Evaluation: Evaluating the quality and the impact of the explanations is another challenge. There is no clear and objective way to measure how good an explanation is, or how much it affects the user’s behavior and satisfaction. Different metrics and methods might be needed for different scenarios and goals.
How Can ChatGPT Plugins Help with Explainability in Recommender Systems?
ChatGPT is a powerful and versatile natural language generation system that can create various types of content, such as text, image, code, etc. ChatGPT also offers a number of plugins that can enable explainable recommender systems, by generating natural language explanations for any text, image, or data. Some of the ChatGPT plugins that can help with explainability are:
- Explain: This plugin can generate natural language explanations for any text, image, or data, by using a combination of natural language understanding, reasoning, and generation. For example, it can explain why a certain movie was recommended, by analyzing the movie’s genre, plot, rating, reviews, etc., and comparing them with the user’s profile, history, mood, etc.
- Similarity: This plugin can measure the similarity between two pieces of text or documents, by using a variety of natural language processing techniques, such as word embeddings, semantic similarity, topic modeling, etc. For example, it can show how similar two movies are, by comparing their text descriptions, keywords, genres, etc.
- Search: This plugin can retrieve documents or snippets similar to a given input text, by using a combination of natural language processing and information retrieval methods, such as query expansion, document ranking, relevance feedback, etc. For example, it can find movies similar to a given movie, by searching a large database of movies and returning the most relevant ones.
These plugins can be easily integrated with any recommender system, by using the ChatGPT API. They can also be customized and fine-tuned for different domains, tasks, and users, by using the ChatGPT interface.
Conclusion
Explainable recommender systems are the systems that provide explanations or justifications for the recommendations they make. They can offer many benefits to the users, such as transparency, trust, personalization, and education. However, they also face many challenges, such as complexity, trade-off, and evaluation. ChatGPT plugins can help with explainability, by generating natural language explanations for any text, image, or data. They can also be easily integrated, customized, and fine-tuned for different scenarios and goals.
ChatGPT Plugins for Social Recommender Systems
What are Social Recommender Systems?
Social recommender systems are systems that leverage the social network and relationships of the users, such as friends, followers, influencers, etc., to provide recommendations. For example, if you are looking for a new book to read, a social recommender system might suggest you some books that your friends or favorite authors have liked or reviewed. Similarly, if you are planning a trip, a social recommender system might recommend you some destinations or hotels that your contacts or travel bloggers have visited or rated.
Social recommender systems aim to improve the quality and diversity of recommendations by incorporating the social information of the users, such as their preferences, opinions, trust, and influence. They also aim to enhance the user experience by making the recommendations more personalized, relevant, and engaging.
Opportunities and Risks of Social Recommender Systems
Social recommender systems offer many opportunities for both users and businesses. For users, social recommender systems can help them discover new products, services, or content that match their interests and needs. They can also help them connect with other users who share similar tastes or goals, and exchange feedback or advice. For businesses, social recommender systems can help them increase customer loyalty, retention, and satisfaction, as well as generate more revenue and profit.
However, social recommender systems also pose some risks and challenges. For users, social recommender systems might expose their personal information or preferences to other users or third parties, which might compromise their privacy or security. They might also influence their decision making or behavior in undesirable ways, such as creating echo chambers, filter bubbles, or herd effects. For businesses, social recommender systems might require more data collection and processing, which might increase the cost and complexity of the system. They might also face ethical or legal issues, such as respecting the user consent, avoiding bias or discrimination, or ensuring fairness and transparency.
ChatGPT Plugins for Social Recommender Systems
ChatGPT is a powerful and versatile AI tool that can generate natural and engaging conversations on various topics and domains. Chat GPT can also be integrated with different platforms and applications, such as social media, e-commerce, or entertainment, to provide interactive and personalized recommendations. ChatGPT plugins are extensions that enable ChatGPT to access and interact with specific platforms or applications, such as Twitter, Reddit, or Instagram. Here are some examples of ChatGPT plugins that can enable social recommender systems:
Twitter Plugin
Twitter is a popular microblogging platform that allows users to post and read short messages, called tweets, that can contain text, images, videos, links, or hashtags. Here Twitter also allows users to follow other users, like or retweet their tweets, or reply to them. Twitter is a rich source of social information, such as user opinions, preferences, sentiments, trends, or events.
The Twitter plugin allows ChatGPT to access and interact with Twitter, such as reading or posting tweets, following or unfollowing users, liking or retweeting tweets, or replying to tweets. The Twitter plugin can also enable ChatGPT to provide social recommendations based on the Twitter data, such as:
- Recommending users to follow based on their interests, preferences, or goals
- Recommending tweets to read or interact with based on their relevance, popularity, or novelty
- Recommending hashtags to use or explore based on their popularity, relevance, or diversity
For example, if a user is interested in learning more about artificial intelligence, the Twitter plugin can recommend them some influential or informative users to follow, such as @AndrewYNg, @ylecun, or @goodfellow_ian. It can also recommend them some tweets that contain useful or interesting information, such as articles, videos, podcasts, or courses. It can also recommend them some hashtags that are related to artificial intelligence, such as #AI, #MachineLearning, or #DeepLearning.
Reddit Plugin
Reddit is a popular online community that allows users to post and discuss various topics, called subreddits, that can range from news, politics, science, gaming, memes, or anything else. The Reddit also allows users to upvote or downvote posts or comments, award them with coins, or reply to them. Reddit is a rich source of social information, such as user opinions, preferences, experiences, or stories.
The Reddit plugin allows ChatGPT to access and interact with Reddit, such as reading or posting posts or comments, upvoting or downvoting posts or comments, awarding or receiving coins, or replying to posts or comments. The Reddit plugin can also enable ChatGPT to provide social recommendations based on the Reddit data, such as:
- Recommending subreddits to join or browse based on their interests, preferences, or goals
- Recommending posts or comments to read or interact with based on their relevance, popularity, or novelty
- Recommending coins to award or receive based on their value, meaning, or impact
For example, if a user is interested in learning more about cooking, the Reddit plugin can recommend them some subreddits to join or browse, such as r/Cooking, r/AskCulinary, or r/FoodPorn. It can also recommend them some posts or comments that contain useful or interesting information, such as recipes, tips, tricks, or reviews. It can also recommend them some coins to award or receive, such as Silver, Gold, or Platinum, to show appreciation or gratitude.
Instagram Plugin
Instagram is a popular photo and video sharing app that allows users to post and view media content, such as photos, videos, stories, or reels, that can contain filters, stickers, captions, or hashtags. The Instagram also allows users to follow other users, like or comment on their posts, or send them direct messages. Instagram is a rich source of social information, such as user preferences, tastes, lifestyles, or inspirations.
The Instagram plugin allows ChatGPT to access and interact with Instagram, such as viewing or posting media content, following or unfollowing users, liking or commenting on posts, or sending or receiving direct messages. The Instagram plugin can also enable ChatGPT to provide social recommendations based on the Instagram data, such as:
- Recommending users to follow based on their interests, preferences, or goals
- Recommending media content to view or interact with based on their relevance, popularity, or novelty
- Recommending filters, stickers, captions, or hashtags to use or explore based on their popularity, relevance, or diversity
For example, if a user is interested in learning more about fashion, the Instagram plugin can recommend them some users to follow, such as @chiaraferragni, @victoriabeckham, or @badgalriri. It can also recommend them some media content that contain useful or interesting information, such as outfits, trends, or tips. It can also recommend them some filters, stickers, captions, or hashtags that are related to fashion, such as #OOTD, #FashionWeek, or #StyleInspo.
Conclusion
Social recommender systems are systems that leverage the social network and relationships of the users to provide recommendations. They offer many opportunities and risks for both users and businesses. ChatGPT is a powerful and versatile AI tool that can generate natural and engaging conversations on various topics and domains. Chat GPT plugins are extensions that enable ChatGPT to access and interact with specific platforms or applications, such as Twitter, Reddit, or Instagram. ChatGPT plugins can enable social recommender systems by providing interactive and personalized recommendations based on the social information of the users.
ChatGPT Plugins for Multi-Criteria Recommender Systems
Have you ever wondered how Netflix, Amazon, or Spotify recommend you the best movies, products, or songs based on your preferences? Well, they use a type of artificial intelligence called recommender systems. Recommender systems are algorithms that analyze the behavior and feedback of users and items, and suggest the most relevant and personalized options for each user. Sounds cool, right?
But wait, there’s more. Recommender systems can also take into account multiple criteria or dimensions of the items and users, such as quality, price, popularity, genre, mood, etc. These are called multi-criteria recommender systems. They can provide more accurate and diverse recommendations, as well as explain the reasons behind them. For example, a multi-criteria recommender system can tell you why you might like a certain movie based on its genre, ratings, reviews, and your previous choices.
Advantages and Disadvantages of Multi-Criteria Recommender Systems
Multi-criteria recommender systems have many advantages over single-criteria recommender systems, such as:
- They can capture the complexity and diversity of user preferences and item features, and provide more relevant and personalized recommendations.
- They can reduce the problem of information overload, by filtering out the items that do not match the user’s criteria or preferences.
- They can increase user satisfaction, trust, and loyalty, by explaining the reasons behind the recommendations and allowing users to provide feedback and adjust their criteria.
- They can improve the performance and scalability of the recommender system, by reducing the sparsity and cold start problems, and using more efficient and robust algorithms.
However, multi-criteria recommender systems also have some disadvantages, such as:
- They can increase the complexity and cost of the recommender system, by requiring more data, computation, and storage resources.
- They can introduce new challenges and trade-offs, such as how to elicit, model, and aggregate the user’s criteria and feedback, how to balance the accuracy and diversity of the recommendations, and how to handle the conflicts and inconsistencies among the criteria.
- They can raise ethical and social issues, such as how to ensure the fairness, transparency, and privacy of the recommender system, and how to avoid the bias, manipulation, and discrimination of the users and items.
ChatGPT Plugins for Multi-Criteria Recommender Systems
If you are interested in building or enhancing your own multi-criteria recommender system, you might want to check out some of the ChatGPT plugins that can enable this functionality. ChatGPT is a powerful and versatile natural language processing framework that can generate and understand natural language texts. It can also integrate with various data sources and platforms, such as web, mobile, social media, etc.
Here are some of the ChatGPT plugins that can help you create a multi-criteria recommender system:
- Rating: a plugin that allows users to rate and review items on a scale of 1 to 5 stars. This plugin can collect and analyze the user’s feedback and preferences, and use them to improve the recommendations. For example, if you use the Rating plugin on your movie recommender system, you can ask the users to rate and review the movies they watch, and use their ratings and reviews to suggest them more movies that they might like.
- Sort: a plugin that allows users to sort and filter items by various criteria, such as price, relevance, popularity, etc. This plugin can help users find the items that match their needs and expectations, and provide them with more options and control. For example, if you use the Sort plugin on your e-commerce recommender system, you can let the users sort and filter the products by their price, category, brand, rating, etc., and show them the most suitable products for them.
- Compare: a plugin that allows users to compare and contrast two or more items based on their features and attributes. This plugin can help users make informed and rational decisions, and provide them with more insights and explanations. For example, if you use the Compare plugin on your music recommender system, you can allow the users to compare and contrast the songs or artists based on their genre, mood, popularity, etc., and tell them why they might prefer one over the other.
These are just some of the ChatGPT plugins that can enable multi-criteria recommender systems. There are many more plugins that can add more functionality and value to your recommender system, such as:
- Recommend: a plugin that generates personalized and relevant recommendations for each user based on their criteria and preferences.
- Explain: a plugin that provides natural language explanations for the recommendations and the criteria used to generate them.
- Feedback: a plugin that collects and responds to the user’s feedback and queries about the recommendations and the recommender system.
- Optimize: a plugin that optimizes and evaluates the performance and quality of the recommender system and the recommendations.
If you want to learn more about these plugins and how to use them, you can visit the ChatGPT website or contact the ChatGPT team. They will be happy to assist you and answer your questions.
ChatGPT Plugins for Deep Learning Recommender Systems
Have you ever wondered how Netflix knows what movies you like? Or how Spotify creates personalized playlists for you? Or how Amazon suggests products that you might be interested in?
If you answered yes to any of these questions, then you have encountered a deep learning recommender system. These are systems that use deep neural networks and other advanced machine learning techniques to learn complex patterns and representations from large and diverse data sources, such as text, images, audio, video, etc., to provide recommendations.
Sounds impressive, right? Well, it is. But it’s not perfect. There are some challenges and limitations that deep learning recommender systems face, such as:
- Data sparsity: There is often not enough data available for each user or item to train a reliable model.
- Data quality: The data may be noisy, incomplete, or biased, which can affect the accuracy and fairness of the recommendations.
- Data privacy: The data may contain sensitive or personal information that needs to be protected from unauthorized access or misuse.
- Computational complexity: The models may be too large or complex to train or deploy efficiently, especially for real-time scenarios.
So, how can we overcome these challenges and improve the performance and user experience of deep learning recommender systems? Well, one possible solution is to use ChatGPT plugins.
ChatGPT plugins are extensions that can enable deep learning recommender systems to interact with users and data in more natural and engaging ways. They can also provide additional functionality and features that can enhance the quality and diversity of the recommendations.
Here are some examples of ChatGPT plugins that can be used for deep learning recommender systems:
Image Plugin
The image plugin allows users to upload and manipulate images, such as cropping, resizing, rotating, etc. This can be useful for scenarios where the user wants to provide visual feedback or preferences to the recommender system, such as:
- Fashion: The user can upload a photo of their outfit and get recommendations for matching accessories or similar styles.
- Art: The user can upload a photo of their favorite painting and get recommendations for other artworks or artists that they might like.
- Travel: The user can upload a photo of their dream destination and get recommendations for flights, hotels, or activities.
Audio Plugin
The audio plugin allows users to upload and manipulate audio files, such as trimming, splitting, merging, etc. This can be useful for scenarios where the user wants to provide auditory feedback or preferences to the recommender system, such as:
- Music: The user can upload a song or a snippet of a song and get recommendations for other songs or artists that they might like.
- Podcasts: The user can upload a podcast or a segment of a podcast and get recommendations for other podcasts or episodes that they might enjoy.
- Audiobooks: The user can upload an audiobook or a chapter of an audiobook and get recommendations for other audiobooks or genres that they might be interested in.
Video Plugin
The video plugin allows users to upload and manipulate video files, such as cutting, editing, adding subtitles, etc. This can be useful for scenarios where the user wants to provide visual and auditory feedback or preferences to the recommender system, such as:
- Movies: The user can upload a movie or a scene of a movie and get recommendations for other movies or genres that they might like.
- TV Shows: The user can upload a TV show or an episode of a TV show and get recommendations for other TV shows or seasons that they might enjoy.
- Games: The user can upload a game or a gameplay of a game and get recommendations for other games or platforms that they might be interested in.
As you can see, ChatGPT plugins can offer a lot of benefits and possibilities for deep learning recommender systems. They can make the interaction more natural, fun, and personalized, and they can also improve the quality and diversity of the recommendations.
Of course, ChatGPT plugins are not the only solution for deep learning recommender systems. There are many other factors and techniques that need to be considered, such as the choice of the model, the optimization of the parameters, the evaluation of the results, etc.
But ChatGPT plugins are definitely a good start. And they are also a good way to showcase the power and potential of ChatGPT, the ultimate AI companion for content creation.
So, what are you waiting for? Try ChatGPT today and unleash your creativity! 😊
ChatGPT Plugins for Reinforcement Learning Recommender Systems
Do you like to learn new things? Doyou enjoy playing games? Do you appreciate feedback? If you answered yes to any of these questions, then you might be interested in reinforcement learning recommender systems.
Reinforcement learning recommender systems are systems that use reinforcement learning, a branch of machine learning that deals with learning from trial and error and rewards and penalties, to optimize the recommendations and interactions with the users.
The Reinforcement learning recommender systems are different from traditional recommender systems, which usually rely on historical data and user preferences to provide recommendations. Reinforcement learning recommender systems can learn from their own actions and the user’s responses, and adapt to the changing environment and user behavior.
Sounds awesome, right? Well, it is. But it’s not easy. There are some challenges and opportunities that reinforcement learning recommender systems face, such as:
- Exploration vs exploitation: The system needs to balance between exploring new options and exploiting the known ones, to avoid getting stuck in a local optimum or missing out on better opportunities.
- Delayed feedback: The system may not receive immediate or clear feedback from the user, which can make it difficult to evaluate the quality of the recommendations and the actions.
- Cold start: The system may not have enough data or knowledge about the user or the items at the beginning, which can limit the quality and diversity of the recommendations.
- User engagement: The system needs to keep the user interested and satisfied, and avoid annoying or boring them with irrelevant or repetitive recommendations.
So, how can we overcome these challenges and improve the performance and user experience of reinforcement learning recommender systems? Well, one possible solution is to use ChatGPT plugins.
ChatGPT plugins are extensions that can enable reinforcement learning recommender systems to interact with users and items in more natural and engaging ways. They can also provide additional functionality and features that can enhance the learning and feedback process.
Here are some examples of ChatGPT plugins that can be used for reinforcement learning recommender systems:
Game Plugin
The game plugin allows users to play and learn from various games, such as chess, tic-tac-toe, sudoku, etc. This can be useful for scenarios where the user wants to have fun and challenge themselves, while also providing implicit feedback to the recommender system, such as:
- Education: The user can play educational games and learn new skills or concepts, while the recommender system can adjust the difficulty and the content based on the user’s performance and progress.
- Entertainment: The user can play entertainment games and enjoy themselves, while the recommender system can recommend new games or genres that the user might like.
- Health: The user can play health games and improve their physical or mental well-being, while the recommender system can monitor the user’s health status and provide personalized advice or interventions.
Quiz Plugin
The quiz plugin allows users to take and create quizzes on various topics and subjects. This can be useful for scenarios where the user wants to test their knowledge and learn new things, while also providing explicit feedback to the recommender system, such as:
- News: The user can take quizzes on current events and stay updated, while the recommender system can recommend new articles or sources that the user might be interested in.
- Books: The user can take quizzes on books and authors and discover new stories, while the recommender system can recommend new books or genres that the user might like.
- Sports: The user can take quizzes on sports and teams and show their fandom, while the recommender system can recommend new matches or leagues that the user might enjoy.
Feedback Plugin
The feedback plugin allows users to provide and receive feedback on the recommendations and the chatbot. This can be useful for scenarios where the user wants to express their opinions and preferences, while also improving the quality and accuracy of the recommender system, such as:
- Movies: The user can provide feedback on the movies and genres that they like or dislike, while the recommender system can provide feedback on the quality and relevance of the recommendations and the chatbot.
- Music: The user can provide feedback on the songs and artists that they like or dislike, while the recommender system can provide feedback on the quality and diversity of the recommendations and the chatbot.
- Food: The user can provide feedback on the food and cuisines that they like or dislike, while the recommender system can provide feedback on the quality and freshness of the recommendations and the chatbot.
As you can see, ChatGPT plugins can offer a lot of benefits and possibilities for reinforcement learning recommender systems. They can make the interaction more natural, fun, and personalized, and they can also improve the learning and feedback process.
Of course, ChatGPT plugins are not the only solution for reinforcement learning recommender systems. There are many other factors and techniques that need to be considered, such as the choice of the model, the optimization of the parameters, the evaluation of the results, etc.
But ChatGPT plugins are definitely a good start. And they are also a good way to showcase the power and potential of ChatGPT, the ultimate AI companion for content creation.
So, what are you waiting for? Try ChatGPT today and unleash your creativity! 😊
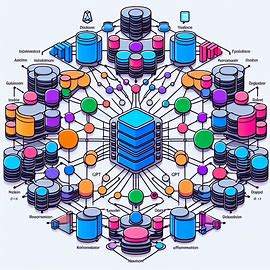
Conclusion
We have reached the end of this blog post, where we explored how ChatGPT plugins can enhance recommender systems. Here We learned what ChatGPT plugins are, how they work, and why they are important for creating personalized and engaging recommendations. We also looked at some examples of ChatGPT plugins for different domains, such as e-commerce, entertainment, and education.
ChatGPT plugins have many benefits for recommender systems, such as:
- They can generate natural and conversational responses that match the user’s tone, style, and preferences.
- They can provide additional information, explanations, or suggestions that complement the recommendations.
- They can handle complex and diverse user queries and feedback, and adapt to the user’s needs and goals.
- They can increase user satisfaction, trust, and loyalty, and reduce churn and bounce rates.
However, ChatGPT plugins also have some challenges, such as:
- They require a lot of data and computational resources to train and run.
- They may not always be accurate, relevant, or appropriate, and may generate misleading or harmful responses.
- They may not respect the user’s privacy, security, or ethics, and may expose sensitive or personal information.
- They may not be compatible with all platforms, devices, or browsers, and may cause technical issues or errors.
Therefore, choosing and using the best ChatGPT plugins for your recommender system is not an easy task. You need to consider several factors, such as:
- The domain and purpose of your recommender system, and the type and size of your data.
- The features and functionalities of the ChatGPT plugins, and the level of customization and control they offer.
- The performance and quality of the ChatGPT plugins, and the metrics and methods to evaluate them.
- The costs and benefits of the ChatGPT plugins, and the trade-offs and risks involved.
To help you with this process, here are some suggestions and tips:
- Do your research and compare different ChatGPT plugins available in the market, and read reviews and testimonials from other users and experts.
- Test and experiment with different ChatGPT plugins on your own data and platform, and see how they work and interact with your users.
- Monitor and analyze the results and feedback from the ChatGPT plugins, and make adjustments and improvements as needed.
- Be transparent and honest with your users about the use and limitations of the ChatGPT plugins, and respect their preferences and choices.
We hope you enjoyed this blog post, and learned something new and useful about ChatGPT plugins and recommender systems. If you have any questions, comments, or suggestions, please feel free to share them with us. We would love to hear from you. 😊
Thank you for reading, and happy recommending! 🙌
aa1a82
7n3ynm
Hey! I just wanted to ask if you ever have any problems with hackers? My last blog (wordpress) was hacked and I ended up losing months of hard work due to no backup. Do you have any methods to stop hackers?
Абсолютно все актуальные события часового мира – трендовые новинки легендарных часовых марок.
Все модели часов от дешевых до экстра роскошных.
https://bitwatch.ru/
Абсолютно все свежие новости часового искусства – свежие коллекции известных часовых компаний.
Абсолютно все коллекции хронографов от бюджетных до супер роскошных.
https://bitwatch.ru/